Mastering AI-Driven Legal Analytics to Outpace Traditional KPIs
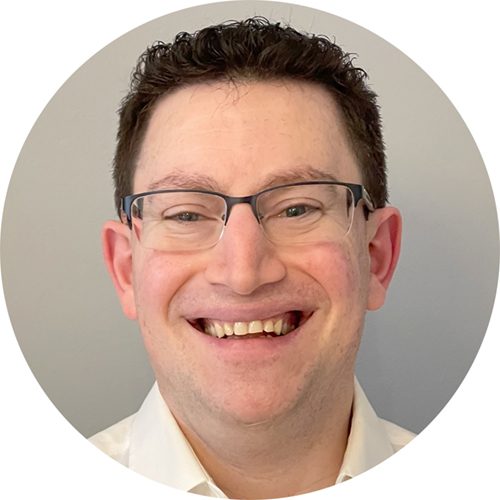
By Colin Levy
March 5, 2025
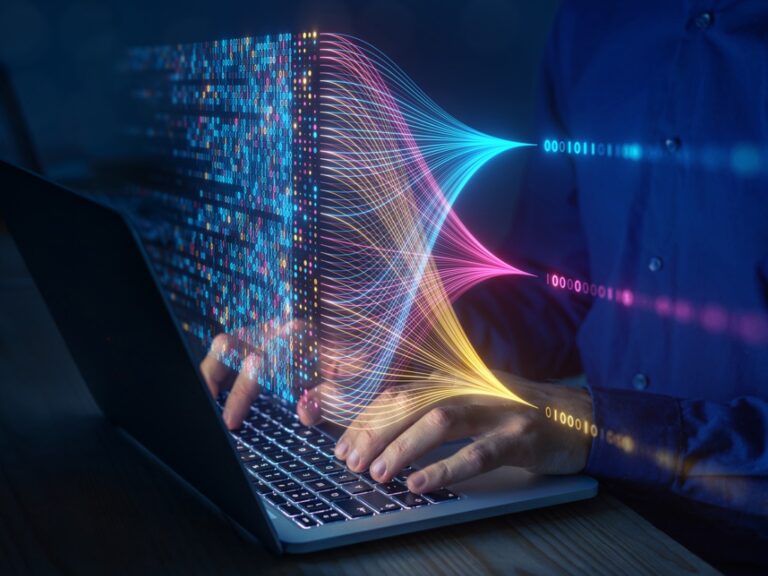
Colin Levy is the author of "The Legal Tech Ecosystem" and the editor of the "Handbook of Legal Tech," serving as a dedicated guide in the legal technology sector. A corporate lawyer by training, he has a passion for innovation and technology. Levy currently serves as the Director of Legal and an Evangelist for Malbek, a provider of enterprise CLM solutions. He also holds roles as both a legal tech startup advisor and investor, remaining deeply connected to the industry's advancements.
Legal teams today are drowning in data but starving for insights with strategic value. Traditional metrics like key performance indicators (KPIs) offer a rearview mirror perspective—documenting what happened but failing to illuminate what’s ahead. Legal departments should move from tracking the past to predicting the future. Enter AI-driven legal analytics: a transformative approach that surfaces insights and drives action.
Transforming Legal Metrics Collection and Analysis
Traditional KPI tracking has long suffered from limitations. Legal operations professionals know the routine well: manually extracting data from multiple systems, wrestling with spreadsheets, and producing static reports that are outdated almost immediately. By the time insights emerge, the opportunity to act has often passed.
AI-powered analytics platforms change this equation. These systems automatically aggregate data from previously disconnected sources—emails, collaboration tools, matter management systems, and billing platforms. The transformation happens beneath the surface, where machine learning algorithms continuously analyze historical patterns while natural language processing extracts meaningful insights from unstructured text.
The impact becomes immediately visible in how legal teams interact with their data. Instead of waiting weeks or months for quarterly reports, operations leaders now view dynamic dashboards that visualize compliance risks, contract bottlenecks, and litigation exposure in real time.
This real-time visibility enables a proactive approach. When contract processing begins slowing down, the system flags the bottleneck immediately. When compliance risks emerge in a particular jurisdiction, alerts trigger before violations occur. Legal operations teams can identify trends as they create corrective actions before issues escalate into crises.
Predictive Analytics
Perhaps the most transformative application of AI in legal operations lies in its predictive capabilities. While traditional KPIs provide historical context, AI-powered analytics illuminate the probable future, fundamentally changing how legal departments approach decision making.
Consider how settlement negotiations traditionally unfold. Attorneys rely on experience, institutional knowledge, and gut feeling to determine whether to settle or litigate. The process, while informed by knowledge, remains subjective and often inconsistent across matters.
AI models trained on historical case data introduce unprecedented objectivity and consistency. These systems analyze thousands of earlier matters, examining judicial rulings, opposing counsel strategies, jurisdictional tendencies, and dozens of other variables. The resulting predictions—often accurate within 70-80% based on proper implementation and training—transform settlement strategy from art to science.
A legal department handling an employment discrimination case might receive a prediction showing a 60% probability of prevailing if litigated, with potential damages ranging between specific dollar amounts if unsuccessful. Armed with this information, attorneys can make data-driven decisions about settlement timing and amounts, often achieving significantly improved outcomes.
Resource allocation similarly transforms through predictive capabilities. Rather than reacting to workload spikes after they occur, legal operations teams can expect them months in advance. The system might predict, based on historical patterns and current matter intake, that the compliance team will face capacity constraints in the third quarter. This foresight enables proactive staffing changes, preventing bottlenecks before they materialize and ensuring consistent service delivery.
Machine Learning for Contract Analysis and Compliance
Contract management represents another area where AI transforms legal operations capabilities. The traditional approach of manual contract review creates persistent challenges: bottlenecks that delay business activities, inconsistent interpretation across reviewers, and the ever-present risk of missing critical terms.
Modern AI contract review tools use natural language processing capabilities to automate what was previously impossible to scale. These systems scan through thousands of contracts to:
- Extract and categorize key clauses automatically, identifying indemnities, termination provisions, and other critical terms
- Identify non-standard, high-risk clauses that deviates from approved templates, flagging potential risks for human review
- Detect compliance gaps, such as missing data protection provisions or outdated regulatory references
- Predict potential issues using sentiment analysis and pattern recognition
For example, some AI platforms may improve accuracy over time through machine learning, potentially reducing manual review by significant percentages (hypothetically 50-70%) while helping ensure adherence to regulatory standards.
AI transforms the narrative of contract management from reactive to proactive. Instead of discovering problematic terms after execution, legal teams identify issues during drafting. Rather than scrambling to address regulatory changes after they take effect, systems track jurisdictions continuously and flag affected agreements automatically. Contract management transforms from an administrative burden to a strategic advantage.
Integration with Legal Operations Systems
The value of AI-driven legal analytics multiplies exponentially when integrated thoughtfully into existing workflows and systems. Legal departments that implement AI understand that technology alone does not drive transformation—it must become seamlessly embedded in how people work every day.
Leading organizations approach this challenge systematically. They begin by mapping current workflows and identifying friction points where AI can add immediate value. They implement centralized operational hubs that unify previously siloed processes like intake, matter management, and reporting. They leverage APIs and no-code interfaces to connect AI capabilities with everyday tools like email, document management systems, and collaboration platforms.
The integration creates a virtuous cycle that compounds benefits. Better data collection enhances AI model accuracy, which improves predictions. Those predictions drive more efficient processes, which generate more comprehensive data. Legal operations professionals work with increasingly intelligent systems that learn from every interaction.
A prime example is automated contract intake and workflow automation—where AI can instantly categorize incoming contracts, route them to the right approver, and predict bottlenecks before they occur. Seamless integration with contract lifecycle management (CLM) platforms ensures contract data is continuously optimized, enabling legal teams to provide real-time insights to procurement, sales, and compliance teams. These metrics feed predictive models that continuously improve resource allocation recommendations. The entire process operates as a seamless, intelligent ecosystem rather than as disconnected tools.
Implementation Roadmap and ROI
Successful implementation of AI-driven legal analytics requires a methodical, phased approach. Organizations that attempt wholesale transformation typically encounter resistance, integration challenges, and underwhelming results. Those that succeed follow a disciplined roadmap that builds momentum through incremental wins.
The journey typically begins with a thorough assessment. Legal operations teams audit data quality across systems, identify high-impact use cases with clear ROI potential, and establish baseline measurements against which to track improvements. This preparation phase, while sometimes overlooked in the enthusiasm to deploy new technology, proves essential for sustainable success.
With foundations established, implementation moves forward through carefully structured pilots. Rather than attempting comprehensive deployment, successful organizations target narrow applications with defined success metrics. A contract review pilot might focus on a single agreement type, such as non-disclosure agreements (NDAs), to show efficiency gains before expanding to more complex agreements.
As pilots show value, strategic scaling follows. The organization expands AI capabilities methodically across more use cases, supported by comprehensive training programs that ensure adoption. Throughout this process, the focus remains on measurable improvements rather than technology for its own sake.
To illustrate potential returns (though actual results vary widely based on implementation specifics), organizations might find that automating contract review reduces attorney time spent by 50-80% for certain agreement types. This efficiency could hypothetically translate to significant annual savings for mid-sized legal departments. Similarly, data-driven settlement strategies might reduce overall resolution costs by meaningful percentages through more accurate case valuation.
These benefits typically compound. As AI systems learn from each interaction, they become increasingly accurate and valuable to the organization. What begins as a tool for efficiency often evolves into a strategic advantage that transforms how legal departments operate.
Ethical and Data Governance Considerations
Implementing AI analytics in legal operations introduces complex ethical considerations that require thoughtful governance. Legal departments bear responsibility given their role in ensuring organizational compliance and ethical conduct.
Bias mitigation represents a central concern. AI systems trained on historical legal data may inadvertently perpetuate existing biases present in that data. A predictive model might recommend settlement strategies that seem statistically sound but reflect historical patterns of unbalanced treatment. Addressing this challenge requires diverse training datasets, regular algorithmic audits, and human oversight of AI-generated recommendations.
Confidentiality presents another critical consideration. Legal departments handle highly sensitive information protected by attorney-client privilege. When implementing third-party AI tools, teams must establish robust data security protocols, including encryption, access controls, and clear data handling procedures. Regular security assessments become essential parts of ongoing governance.
Appropriate human oversight forms the third pillar of ethical AI implementation. While automation drives efficiency, attorneys must maintain supervision of AI outputs to meet ethical standards of competence and accountability. This balance requires thoughtfully designed workflows where AI augments rather than replaces professional judgment.
Organizations that navigate these considerations typically establish formal governance frameworks that balance innovation with safeguards. These frameworks include clear policies for data handling, regular auditing of AI outputs, and maintained human oversight of critical decisions. The most effective approaches integrate ethical considerations from the beginning rather than addressing them as afterthoughts.
Charting the Future of Legal Operations Analytics
The journey from traditional KPIs to AI-driven analytics represents a fundamental shift in how legal departments operate. Rather than merely documenting past performance, leading organizations now predict future outcomes and optimize. This transformation elevates legal operations from cost center to strategic business partner.
Success in this new paradigm requires thoughtful alignment of technology, process, and people. Nowhere is this clearer than in contract management, where AI-driven insights shift legal teams from reactive to proactive. By embedding AI into CLM workflows, organizations gain an unprecedented ability to forecast risk, accelerate deal velocity, and drive strategic value. AI isn’t just enhancing legal analytics—it’s redefining how businesses manage contracts, mitigate risks, and seize opportunities.
As AI platforms continue to mature, legal operations teams that thoughtfully integrate these capabilities will deliver unprecedented value. They will decide based on data rather than intuition, manage risks proactively rather than reactively, optimize resources precisely rather than generally, and collaborate seamlessly rather than sporadically.
The future belongs to legal departments that embrace this transformation. By advancing beyond traditional KPIs to predictive, AI-driven insights, they position themselves not just as efficient operators but as strategic partners capable of delivering measurable, scalable value to the entire organization.
Critical intelligence for general counsel
Stay on top of the latest news, solutions and best practices by reading Daily Updates from Today's General Counsel.
Daily Updates
Sign up for our free daily newsletter for the latest news and business legal developments.